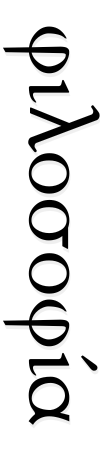
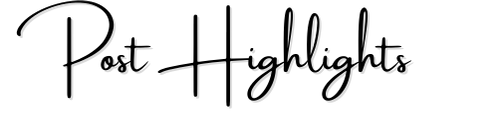
- “High-quality AI systems begin by gathering diverse, comprehensive, and relevant datasets. This means including data from a variety of sources to cover as much variation in the input as possible, which helps in developing a more versatile and robust model.“
- “Proper data cleaning helps in reducing noise and improving the accuracy of the model.“
- “Accuracy in data annotation and labeling is critical because any mistake in labeling can lead to incorrect learning by the model.“
- “Ensuring the ethical sourcing of data and actively working to identify and mitigate biases in the dataset are fundamental to developing fair and responsible AI.“
- “To enhance the quality and quantity of training data, AI developers often use data augmentation techniques.“
- “Avoiding the recursive reinforcement of inferior content in AI systems is crucial for maintaining quality, fairness, and relevance.“
- “Employing techniques specifically designed to detect and mitigate biases in AI models is crucial.“
Table of Contents: (Click any link below to navigate to that section.)
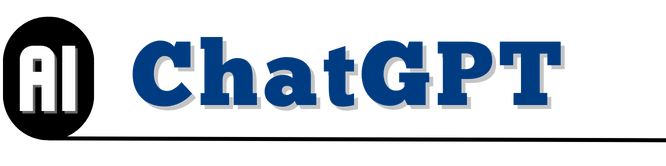
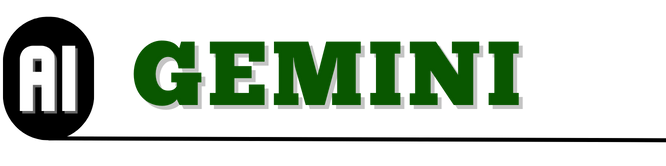
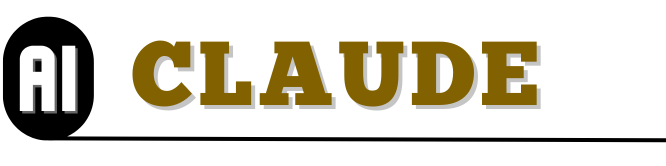
How do AI platforms ensure their training data is of the highest quality?
Ensuring the highest quality of training data for AI platforms involves several strategic steps. These steps can significantly impact the effectiveness and fairness of the AI models developed. Here’s a breakdown:
- Data Collection: The foundation of any AI model is robust data collection. High-quality AI systems begin by gathering diverse, comprehensive, and relevant datasets. This means including data from a variety of sources to cover as much variation in the input as possible, which helps in developing a more versatile and robust model.
- Data Cleaning and Preprocessing: Once data is collected, it needs to be cleaned and preprocessed. This step involves removing errors, duplicates, and irrelevant information from the dataset. It also includes normalizing data (bringing it to a standard format) and handling missing values. Proper data cleaning helps in reducing noise and improving the accuracy of the model.
- Data Annotation and Labeling: For supervised learning models, data annotation and labeling are crucial. This process involves tagging the collected data with the correct labels or outcomes. Accuracy in this step is critical because any mistake in labeling can lead to incorrect learning by the model. Many organizations employ expert annotators and use multiple checks to ensure the labels are accurate.
- Quality Checks: Continuous quality checks are essential to maintain the integrity of the training data. This involves statistical analyses to ensure that the data distribution is balanced and representative of the real-world scenarios the AI model will encounter. It also includes checking for biases in the data to prevent them from being learned by the model.
- Ethical Considerations and Bias Mitigation: Ensuring the ethical sourcing of data and actively working to identify and mitigate biases in the dataset are fundamental to developing fair and responsible AI. This might involve including data from underrepresented groups, using techniques to balance the data, and regularly auditing the model’s decisions for fairness and accuracy.
- Data Augmentation: To enhance the quality and quantity of training data, AI developers often use data augmentation techniques. This can include artificially creating new training examples through modifications of existing data, such as rotating images in computer vision tasks or synonym replacement in text. Augmentation helps in making the model more robust to variations it might face in the real world.
- Continuous Monitoring and Updating: AI platforms often continuously monitor the performance of their models and the quality of their training data. This involves updating the datasets with new, relevant data over time and retraining the models to adapt to changes in the data distribution or to improve performance.
- Legal and Compliance Checks: Ensuring that data collection and use comply with all relevant laws and regulations, including privacy laws and data protection regulations, is crucial. This often involves anonymizing personal data and obtaining the necessary permissions for data use.
By carefully implementing these steps, AI platforms strive to ensure that their training data is of the highest quality, which is crucial for developing effective, fair, and responsible AI models.
Ensuring high-quality training data is crucial for AI platforms, as it directly impacts the model’s performance and reliability. Here are some key methods AI platforms use to achieve this:
- Defining Quality Metrics: First, they establish what “high-quality” means for their specific project. This involves understanding the desired functionality of the AI and creating clear criteria for data labeling and selection.
- Data Collection and Cleaning: Data is carefully collected from reliable sources to minimize bias and errors. Techniques like data cleaning and normalization ensure consistency in format and units throughout the dataset.
- Human Annotation: A crucial step is labeling the data. AI platforms often leverage human annotators to assign labels to data points. To ensure consistency, multiple annotators might review the same data, and disagreements are resolved through a consensus process.
- Data Review and Validation: The labeled data undergoes rigorous review by data scientists or domain experts. This helps identify and rectify errors or inconsistencies in labeling. Techniques like using “gold sets” – pre-vetted data with established labels – can be used for comparison.
- Addressing Bias: AI platforms are aware of the potential for bias in training data. They might employ techniques to de-bias the data or balance the dataset to include a wider range of examples.
- Automated Quality Checks: While human review is vital, AI platforms can leverage automated tools to scan for common errors and inconsistencies, improving efficiency.
AI platforms employ several techniques to ensure the quality of their training data:
- Data Curation: They have teams of human annotators who carefully curate and label the data used for training AI models. This involves tasks like removing offensive, biased or low-quality content from the datasets.
- Data Filtering: Advanced data filtering techniques are used to automatically remove noisy, duplicate or irrelevant data points from the training sets based on certain rules and quality checks.
- Data Augmentation: Processes like translation, paraphrasing, spelling modifications etc. are used to synthetically augment and diversify the training data without introducing low-quality inputs.
- Multi-task Learning: AI models are trained on a variety of tasks simultaneously using shared representations, which helps the model learn more robust features applicable across domains.
- Human Oversight: There is continuous human monitoring and evaluation of the model outputs during training to identify issues and refine the data accordingly.
- External Audits: Reputable AI companies often engage third-party auditors to evaluate their training practices, model behavior and identify potential risks/biases.
- Testing: Extensive testing of the models on held-out evaluation datasets is done to measure performance and uncover any training data deficiencies.
The quality of training data is crucial for developing safe, unbiased and performant AI systems aligned with human values and societal expectations. Leading AI labs invest significant resources into curating high-quality training corpora.
What are the actual sources of training data?
The sources of training data for AI models are varied and numerous, reflecting the wide range of applications and needs in the field. Here’s a look at some of the most common sources:
- Public Datasets: There are countless public datasets available for a variety of domains such as healthcare, finance, natural language processing, and computer vision. These datasets are often provided by academic institutions, government agencies, and non-profit organizations. Examples include the ImageNet database for image recognition tasks, the UCI Machine Learning Repository, and datasets provided by the U.S. Government’s open data initiative.
- Web Scraping: The internet is a vast source of data, and web scraping is a technique used to extract data from websites. This can include text, images, and more. It’s commonly used for gathering datasets for natural language processing tasks, sentiment analysis, and competitive analysis.
- Social Media and Online Platforms: Data from social media platforms and other online forums can be invaluable, especially for tasks related to sentiment analysis, trending topics, and consumer behavior. This includes data from Twitter, Facebook, Reddit, and other platforms where users generate content.
- Sensors and IoT Devices: For applications related to real-world physical phenomena, such as weather forecasting, autonomous vehicles, and smart homes, data from sensors and IoT (Internet of Things) devices are crucial. These can include temperature sensors, cameras, GPS devices, and more.
- Generated Synthetic Data: When real data is scarce or privacy concerns restrict the use of real-world data, synthetic data generation can be a solution. This involves creating artificial data that mimics the statistical properties of real datasets. It’s useful for training models in sensitive domains like healthcare where patient confidentiality is paramount.
- Corporate Data: Many companies use their internal data as a source for training AI models. This can include customer transaction data, interaction logs, sales records, and more. This data is particularly valuable for tasks like personalized recommendation systems, customer service automation, and operational optimization.
- Crowdsourcing and Annotated Datasets: Platforms like Amazon Mechanical Turk or specialized data annotation companies allow for the collection and annotation of data by humans. This is especially useful for tasks that require understanding of context or subjective judgment, like sentiment analysis or categorizing images based on content.
- Government and Administrative Records: Data from government agencies, such as census data, health records, and economic indicators, can be used for a wide range of applications including public policy research, urban planning, and healthcare studies.
- Academic and Research Institutions: Universities and research organizations often produce and share datasets related to their studies. These datasets are typically well-documented and cover a wide range of topics, from linguistics to astrophysics.
- Partnerships and Collaborations: Companies and research institutions sometimes form partnerships to share data in a way that benefits all parties involved. This can provide access to unique datasets that would otherwise be unavailable or difficult to compile.
The choice of data source depends on the specific requirements of the AI project, including the domain of application, the volume of data needed, and considerations related to privacy and data sensitivity.
Training data for AI platforms comes from a variety of sources, depending on the specific project and the type of data needed. Here’s a breakdown of some common sources:
- Open-source Datasets: These are publicly available collections of data on various topics, often curated by research institutions or organizations. Platforms like Kaggle and Google Dataset Search offer a vast resource of open-source training data.
- Web Scraping: Extracting data from websites and online sources can be a valuable technique, particularly for text-based AI projects. This requires careful consideration of copyright and ethical harvesting practices.
- Curated Datasets: Companies specializing in data collection and curation can provide high-quality, pre-labeled datasets tailored to specific AI applications.
- Custom Data Collection: For specialized tasks, AI platforms might collect their own data. This could involve gathering customer support logs, sensor readings, or video footage, which is then annotated for the specific project.
AI platforms typically source their training data from a variety of places, including:
- Web Crawling: Large language models ingest massive amounts of text data crawled from the internet, including websites, online books, articles, forums, and social media.
- Digital Libraries: They leverage large digital libraries, databases, and repositories containing books, academic papers, news archives, etc.
- Proprietary Datasets: Companies often have access to proprietary datasets stemming from their own products/services like search queries, user interactions, conversations etc.
- Public Datasets: There are many open-source datasets created by researchers, governments, non-profits etc. for various NLP/CV tasks.
- Licensed Data: AI companies also license and purchase high-quality annotated datasets from data marketplaces and providers.
- Partnerships: Collaborations with other companies, organizations and institutions to securely access their data sources.
- Crowdsourcing: Crowdsourced annotations from internet workers on platforms like Mechanical Turk for data labeling tasks.
- Audio/Video: Audio and video data from sources like podcasts, movies, TV shows, instructional videos etc. can provide rich multi-modal training signals.
The data is usually processed through filtering, deduplication, cleaning and safeguarding steps before being used for model training. There is an increasing focus on having diverse, representative and fully-documented data sources to develop unbiased and robust AI systems.
How do AI experts avoid a recursive reinforcement of inferior content?
Avoiding the recursive reinforcement of inferior content in AI systems, particularly in those that generate or recommend content, is crucial for maintaining quality, fairness, and relevance. Here are several strategies that AI experts employ to prevent this cycle:
- Diverse and Representative Training Data: Ensuring that the training data is diverse and representative of a broad range of perspectives helps prevent the model from overfitting to a narrow set of viewpoints or content types. This involves actively seeking out and including data from underrepresented groups and diverse sources.
- Regular Model Evaluation and Updating: AI systems should be regularly evaluated against new, unseen data to ensure they continue to perform well and do not perpetuate or amplify biases. Regular updates to the model, including retraining with new and diverse data sets, can prevent the reinforcement of inferior content.
- Bias Detection and Mitigation Techniques: Employing techniques specifically designed to detect and mitigate biases in AI models is crucial. This might involve algorithmic approaches to reduce bias in the training data or in the model’s predictions, as well as the use of fairness metrics to evaluate model outcomes across different groups.
- User Feedback Loops: Incorporating feedback mechanisms where users can report inappropriate or low-quality content helps in identifying and correcting instances where the model may reinforce such content. This feedback can be used to adjust the model’s behavior or to flag content for review.
- Content Diversity Algorithms: Implementing algorithms that explicitly aim to increase the diversity of content being recommended or generated can help counteract the tendency towards reinforcing popular or narrow content types. These algorithms can introduce variety and expose users to a wider range of content.
- Ethical and Responsible AI Practices: Adopting a set of ethical guidelines and responsible AI practices that include considerations for content quality, fairness, and the potential social impact of AI recommendations. This often involves multidisciplinary teams that include ethicists, sociologists, and domain experts alongside AI developers.
- Transparency and Explainability: Making AI systems transparent and explainable helps users and developers understand why certain content is being recommended or generated. This understanding can be crucial for identifying when inferior content is being reinforced and for making necessary adjustments.
- Content Moderation: Implementing content moderation systems, either automated, human, or a combination of both, helps in filtering out low-quality, harmful, or irrelevant content before it reaches users. This is particularly important in platforms where user-generated content is prevalent.
- Multi-Dimensional Recommendation Metrics: Moving beyond simple engagement metrics (like clicks or views) to evaluate content. Incorporating a range of metrics that assess content quality, relevance, diversity, and user satisfaction provides a more holistic approach to content recommendation and generation.
- Collaborative Filtering with Caution: While collaborative filtering is a popular technique for recommendation systems, relying solely on it can lead to echo chambers where similar content is continuously recommended. Balancing this with content-based filtering and other strategies can help mitigate such issues.
By implementing these strategies, AI experts work to ensure their models and systems are not just technically proficient but also socially responsible, providing users with high-quality, diverse, and relevant content.
Recursive reinforcement of inferior content, also known as echo chambers or feedback loops, is a major concern in AI training data. Here are some ways AI experts tackle this issue:
- Data Augmentation and Variation: AI experts can manipulate existing data to create variations, increasing the diversity of the training set and reducing reliance on any one source. Techniques like text paraphrasing, image rotation, or audio noise injection can be used.
- Active Learning: This approach involves the AI model itself guiding the data collection process. The model identifies areas where its training is lacking and requests more data that specifically addresses those weaknesses. This helps steer the training away from prevalent biases in the existing data.
- Transfer Learning and Pre-trained Models: Leveraging pre-trained models on a massive dataset can provide a strong foundation for new AI projects. The AI can then be fine-tuned on a smaller, more targeted dataset, reducing the influence of any biases within that specific dataset.
- Human-in-the-Loop Training: Involving human experts in the training loop helps identify and remove low-quality or biased data before it significantly impacts the model. This can involve setting up validation stages where humans review the model’s performance and correct its course if bias creeps in.
- Metric Monitoring and Bias Detection: AI experts constantly monitor the AI model’s performance for signs of bias. This might involve analyzing the model’s outputs across different demographics or using fairness metrics to identify disparities. Once detected, corrective actions can be taken to mitigate bias.
AI experts employ several techniques to avoid recursive reinforcement and propagation of inferior content during model training:
- Careful Data Curation
Human experts meticulously review and filter out low-quality, biased, offensive or factually incorrect content from the training data corpus before it is ingested by the model. - Diverse Data Sources
They ensure the training data comes from a wide variety of reputable sources representing different perspectives, domains and styles to minimize bias amplification from any single source. - Data Monitoring
Ongoing monitoring of the model outputs during training to detect concerning patterns, hallucinations or regressions which could signal propagation of unwanted content. - Iterative Refinement
If inferior outputs are identified, the human curators revisit the corresponding training samples to remove or correct the problematic data points before continuing training. - Controlled Environments
Some models are trained in controlled synthetic environments using curated data rather than unconstrained internet crawls to avoid ingesting noisy web content. - Multi-task training
Training on a diverse set of tasks using shared representations acts as a regularizer preventing over-specialization on any single inferior domain. - Human Feedback
Getting human ratings and feedback on sample model outputs which is then incorporated back into the training process. - Ensemble Methods
Combining outputs from multiple models trained on different subsets can help cancel out individual model biases.
The key is maintaining a tight human feedback loop and putting robust curation practices in place to prevent runaway reinforcement of low-quality patterns during the AI training process.
Create a 7-item quiz on the entire thread above.
Quiz Questions
- What is the first step in ensuring the highest quality of training data for AI platforms?
- Which technique is used to extract data from websites, contributing to AI training data sources?
- What type of data is crucial for applications related to real-world physical phenomena, such as autonomous vehicles and smart homes?
- How can synthetic data be useful in AI model training?
- What is a common strategy to prevent AI models from perpetuating or amplifying biases?
- Why is it important for AI systems to include mechanisms for user feedback?
- What role does content moderation play in preventing the recursive reinforcement of inferior content in AI systems?
Quiz Answers
- Data Collection
- Web Scraping
- Data from sensors and IoT (Internet of Things) devices
- When real data is scarce or privacy concerns restrict the use of real-world data
- Employing techniques specifically designed to detect and mitigate biases
- It helps in identifying and correcting instances where the model may reinforce inappropriate or low-quality content.
- It helps in filtering out low-quality, harmful, or irrelevant content before it reaches users.
AI Training Data Quiz
- What is a crucial first step AI developers take to ensure high-quality training data?
- How can AI platforms leverage human expertise to improve the accuracy of training data?
- Besides human review, what automated tools can be used to enhance training data quality?
- When building an AI for image recognition, where might a developer acquire training data?
- What strategy can help AI models identify and address weaknesses in their training data?
- How can pre-trained models help mitigate bias in a new AI project?
- Besides monitoring the model’s outputs, what other technique can AI experts use to detect bias in a trained model?
Answers:
- Defining Quality Metrics
- Human Annotation
- Automated Quality Checks
- Open-source Datasets or Web Scraping
- Active Learning
- Transfer Learning
- Fairness Metrics
- What is one technique AI platforms use to ensure the quality of their training data?
- Name one common source of training data for AI models.
- True or False: AI experts only use training data from the internet to avoid potential biases.
- What is a potential risk that AI experts aim to avoid when training models?
- Which of the following is NOT a method used to prevent recursive reinforcement of inferior content?
A) Careful data curation
B) Using only data from trusted sources
C) Iterative refinement
D) Training models entirely without human oversight - Why is it important to have diverse data sources when training AI models?
- What role does human feedback play in preventing the propagation of low-quality content during AI training?
Answers:
- Data curation, data filtering, data augmentation, multi-task learning, human oversight, external audits, testing.
- Web crawling, digital libraries, proprietary datasets, public datasets, licensed data, partnerships, crowdsourcing, audio/video data.
- False
- Recursive reinforcement of inferior content
- D) Training models entirely without human oversight
- To minimize bias amplification from any single source and have representative data.
- Human feedback on sample outputs is incorporated back into the training process to identify and correct issues.
Provide 15 discussion questions relevant to the content above.
- How does the diversity of training data impact the performance and fairness of AI models?
- Discuss the ethical implications of web scraping for collecting AI training data.
- How do sensor and IoT device data transform the capabilities of AI in understanding and interacting with the physical world?
- What are the challenges and benefits of using synthetic data in AI training?
- Explore the significance of bias detection and mitigation techniques in the development of fair AI systems.
- How can user feedback loops be effectively implemented in AI systems to improve content quality?
- In what ways do diverse and representative training datasets prevent the reinforcement of stereotypes by AI models?
- Discuss the role of regular model evaluation and updating in maintaining the relevance and accuracy of AI systems.
- What are the potential risks and ethical considerations associated with using corporate data for training AI models?
- How do content diversity algorithms contribute to the variety and fairness of AI-generated content?
- Evaluate the impact of transparency and explainability in AI systems on user trust and understanding.
- Discuss the importance of multidisciplinary teams in the development of ethical and responsible AI practices.
- How does content moderation influence the user experience and content quality in platforms powered by AI?
- Explore the implications of using collaborative filtering in recommendation systems and its potential to create echo chambers.
- Discuss how AI platforms can balance the trade-off between personalization and privacy in collecting and using training data.
Discussion Questions on AI Training Data:
- Bias vs. Accuracy: Can striving for perfectly unbiased training data ever come at the expense of accuracy in certain AI applications? Why or why not?
- Data Privacy Concerns: How can AI developers ensure responsible data collection practices that respect user privacy when scraping data from the web?
- Who Owns the Data? As AI becomes more prevalent, who should have ownership rights over the data used to train these models?
- Regulation vs. Innovation: Should there be stricter regulations on how AI companies obtain and use training data, or could this stifle innovation?
- The Human Factor: How can we ensure that human bias isn’t unintentionally introduced during data annotation and review stages?
- Evolving Standards: As AI technology advances, how will the definition of “high-quality training data” need to adapt and change?
- The Cost of Good Data: High-quality training data collection and curation can be expensive. How can we ensure access to good data for smaller AI developers and startups?
- Synthetic Data Generation: Can synthetically generated data be a viable alternative to real-world data for training certain AI models?
- Explainability vs. “Black Box” Models: If an AI model achieves impressive results but the training data is opaque, is it still a responsible approach?
- The Future of Open Data: How can we encourage more open-source data sharing to accelerate responsible AI development?
- Global Considerations: How can we ensure that training data reflects the diversity of cultures and languages to avoid regional biases in AI models?
- Human Oversight vs. Automation: When does it become more efficient to rely on automated quality checks for training data, versus involving human experts?
- The Ethics of Active Learning: Could relying on active learning models to guide data collection potentially lead to the reinforcement of existing biases?
- Measuring Fairness: What are the limitations of using fairness metrics to detect bias in AI models, and what alternative approaches can be considered?
- The Long Game: How can we ensure that ongoing monitoring and bias detection remain priorities as AI models are continuously updated and refined?
Here are 15 discussion questions relevant to ensuring high-quality training data for AI models:
- What are some potential sources of bias in training data, and how can they be mitigated?
- How can human annotators and curators be trained and incentivized to provide high-quality data labeling?
- What are the trade-offs between using web-crawled data versus more curated, proprietary datasets?
- How can synthetic data augmentation techniques be improved to better capture real-world diversity?
- What role should external auditors play in evaluating AI training practices and datasets?
- How can we develop better benchmarks and evaluation metrics for assessing training data quality?
- What are the unique challenges in curating high-quality audio, video, and multimodal training data?
- How can we ensure proper consent and privacy when using proprietary user data for AI training?
- What are the risks of overly sanitizing or constraining training data to the point of lacking real-world relevance?
- How can we incentivize companies and organizations to share high-quality data for AI research?
- What techniques can be used to detect and mitigate recursive reinforcement of unwanted patterns during training?
- How should we balance the need for transparency around training data sources with privacy and intellectual property concerns?
- What role can crowdsourcing and citizen science efforts play in curating large, diverse datasets?
- How can we develop AI training practices that are more interpretable and amenable to human oversight?
- What are some emerging sources of training data (e.g. IoT devices, AR/VR, robotics) and their associated challenges?
Table of Contents: (Click any link below to navigate to that section.)
- How do AI platforms ensure their training data is of the highest quality?
- What are the actual sources of training data?
- How do AI experts avoid a recursive reinforcement of inferior content?
- Create a 7-item quiz on the entire thread above.
- Provide 15 discussion questions relevant to the content above.
Leave a comment